According to the British Social Attitudes Survey held in 2022, the most common reason why people were dissatisfied with the NHS was because of long GP and hospital waiting times. In this article we’ll discuss how data analysis in healthcare can be used to reduce patient wait times and improve overall patient satisfaction.
What is data analytics in healthcare?
Data analytics is becoming a critical component of healthcare. Data analysis in healthcare refers to the process of examining and interpreting large sets of healthcare data. It involves using statistical techniques to identify patterns, trends, and insights that can inform decision making and improve patient outcomes.
This data can come from various sources such as electronic health records, insurance claims, clinical trials, and patient-generated data. The purpose of data analysis in healthcare is to help healthcare organisations better understand their patient population and optimise their care delivery processes.
The importance of data in healthcare
By gathering hospital data insights, healthcare providers can identify areas where they can improve patient care, reduce costs, and increase efficiency. Examples of how data analysis is used in healthcare include identifying trends in disease prevalence, predicting patient readmissions, and optimising medication dosages. With the help of data analysis, healthcare providers can make evidence-based decisions and provide more personalised care to their patients.
How do A&E departments currently use data?
The A&E process begins when a patient arrives at the hospital with a medical emergency. The patient is initially assessed by a triage nurse who assigns them to a priority category based on the severity of their condition. This information is recorded in the hospital's electronic health record system, along with other relevant patient information such as medical history, allergies, and medications.
Once the patient is seen by a physician or other medical professional, additional data is collected, including diagnostic test results and treatment information. All of this data is entered into the hospital's electronic health record system, creating a comprehensive patient record that can be used for analysis and quality improvement purposes.
What are some common congestion issues within the NHS or healthcare services currently?
So why are NHS wait times so long? Below are some congestion issues healthcare services currently face:
-
Negative impact from COVID-19: By March 2022, there were more than 300,000 people waiting more than a year for routine planned care, compared to only 1,600 people in February 2020 before services were substantially affected by Covid-19. The Covid-19 pandemic has had a huge impact on NHS wait times.
-
Staffing shortages: A&E departments are often understaffed, leading to longer wait times for patients and reduced quality of care.
-
Limited capacity: Many A&E departments have limited capacity, which can lead to overcrowding and longer wait times.
-
Inappropriate use of A&E: Patients visit A&E departments for minor ailments that could be treated elsewhere or even at home.
-
Delays in test results: Delays in diagnostic test results can lead to longer wait times for patients and congestion in the A&E department.
-
Delayed discharges: When patients are medically fit to be discharged but are unable to leave the hospital due to a lack of available community care services. This can lead to further congestion at the end of the A&E process.
Addressing these congestion issues is critical to improving patient outcomes and reducing the strain on A&E departments. Identifying the root causes of congestion means that healthcare providers can implement targeted solutions.
The impact of wait times on patient outcomes and satisfaction
Here are some of the ways in which long wait times can negatively impact patients:
- Delayed treatment: Patients with serious conditions such as heart attacks or strokes may experience even worse outcomes if treatment is delayed.
- Increased anxiety: Long wait times can cause patients to become anxious and stressed, which can have a negative impact on their overall well-being.
- Reduced satisfaction: Longer wait times often lead to dissatisfied patients, lower patient retention rates and damage the reputation of healthcare providers.
- Increased costs: Long wait times can result in increased healthcare costs as patients may require additional care or treatments due to delayed treatment.
- Higher readmission rates: Patients who experience long wait times may be more likely to experience complications or require readmission, leading to higher readmission rates and increased healthcare costs
RECOMMENDED READING: 
Techniques for streamlining patient flow and reducing wait times
How can healthcare providers use hospital data insights to improve waiting times? Learn more with these examples below:
Predictive analytics
Using predictive analytics in healthcare can be an effective way to improve patient wait times. Predictive analytics tools can help healthcare providers accurately forecast patient demand, resource utilisation, and staffing needs. This, in turn, leads to the optimisation of operation procedure, reduction in wait times, and improvement in patient experience.
For example, hospitals can use predictive analytics to forecast patient arrivals and adjust staffing levels accordingly. This ensures that they have the appropriate number of clinicians and resources available to meet patient demand. By analysing patient data and predicting which patients are likely to experience longer wait times, hospitals can proactively triage and prioritise care based on urgency.
Real-time monitoring
By using technology such as real-time location systems (RTLS), hospitals can monitor the flow of patients and resources in real-time. This allows them to quickly identify constraints and adjust their operations accordingly. For example, if a particular area of the hospital is experiencing a high volume of patients, the hospital can use real-time monitoring to quickly deploy additional staff or resources to that area.
Real-time monitoring can also help hospitals identify when patients are waiting longer than expected and trigger alerts for staff to take action. Real-time monitoring can also help hospitals spot trends over time and adjust their operations accordingly, helping to continually improve the patient experience and reduce wait times.
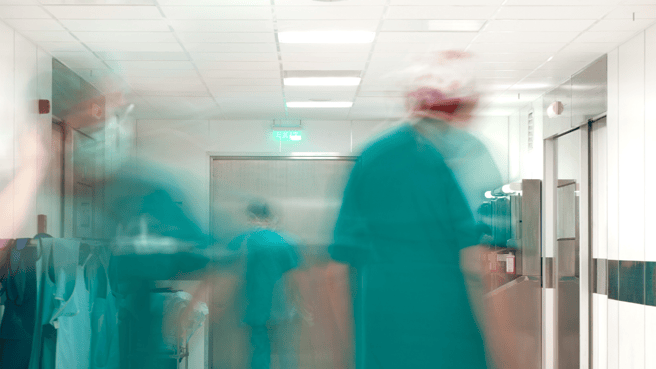
Analysing resource allocation
Analysing data of resource allocation in hospitals can significantly improve patient wait times by providing insights into how resources are being used and where. With this data, hospitals can:
- Better predict patient demand
- Allocate staff and resources accordingly
- Streamline processes; and
- Monitor performance to ensure that patients receive timely care.
For example, hospitals can use data to identify the busiest periods and allocate more staff during those times, reducing wait times and improving patient satisfaction. By optimising resource allocation and streamlining processes, hospitals can also increase efficiency and productivity, allowing them to see more patients and reduce wait times even further.
Identification of high-risk patients
By prioritising high-risk patients, hospitals can allocate resources and schedule appointments more effectively, reducing wait times for these patients while also freeing up resources for other patients. Additionally, early intervention for high-risk patients can prevent emergency department visits and hospitalisations, which can lead to longer wait times for all patients. By effectively triaging and prioritising patients based on risk, healthcare providers can ensure that all patients receive the appropriate levels of care they need.
Challenges and considerations when using data analytics in A&E
Below are challenges that healthcare providers may face when using data:
- Privacy and security concerns - healthcare data is subject to strict privacy regulations e.g GDPR and healthcare providers must ensure that patient data is protected from unauthorised access or breaches.
- Difficult data integration - healthcare data is often stored in multiple systems and formats, making it difficult to integrate and analyse effectively.
- Data quality - The accuracy and completeness of hospital data insights can vary. Incomplete or incorrect data can lead to inaccurate analysis and decision making.
- Inadequate staff training - Without proper training, healthcare providers may struggle to effectively collect, manage, and analyse healthcare data. Proper data upskilling means that healthcare workforces can use data results to their full potential, ultimately improving patient care.
Future trends in data analytics in healthcare
- Artificial Intelligence - AI-powered triage systems can help A&E departments to quickly identify patients who require urgent care and prioritise them for treatment. This can reduce wait times and improve patient outcomes.
- Wearable technology - Wearable technology, such as smartwatches and fitness trackers, can provide A&E departments with real-time patient data, enabling them to proactively manage patient health and prevent emergency visits.
- Remote monitoring - Remote monitoring technologies can enable A&E departments to monitor patients who have been discharged from the hospital, reducing readmission rates and improving patient care.
NHS-tailored Cambridge Spark apprenticeships
At Cambridge Spark we deliver government-funded, tailored data and AI apprenticeships that provide the NHS workforce with the skills needed to reshape healthcare services. Our apprenticeships offer learners the chance to develop valuable skills, from building data literacy in Excel, through to earning a MSc-level qualification in artificial intelligence. By completing an NHS-tailored apprenticeship, learners can have real impact within their organisations. For example, by automating reporting processes in Python, apprentices can achieve 99% less time spent on reporting and 77% fewer dropped operations.
Discover our data apprenticeships available to professionals across all industries.