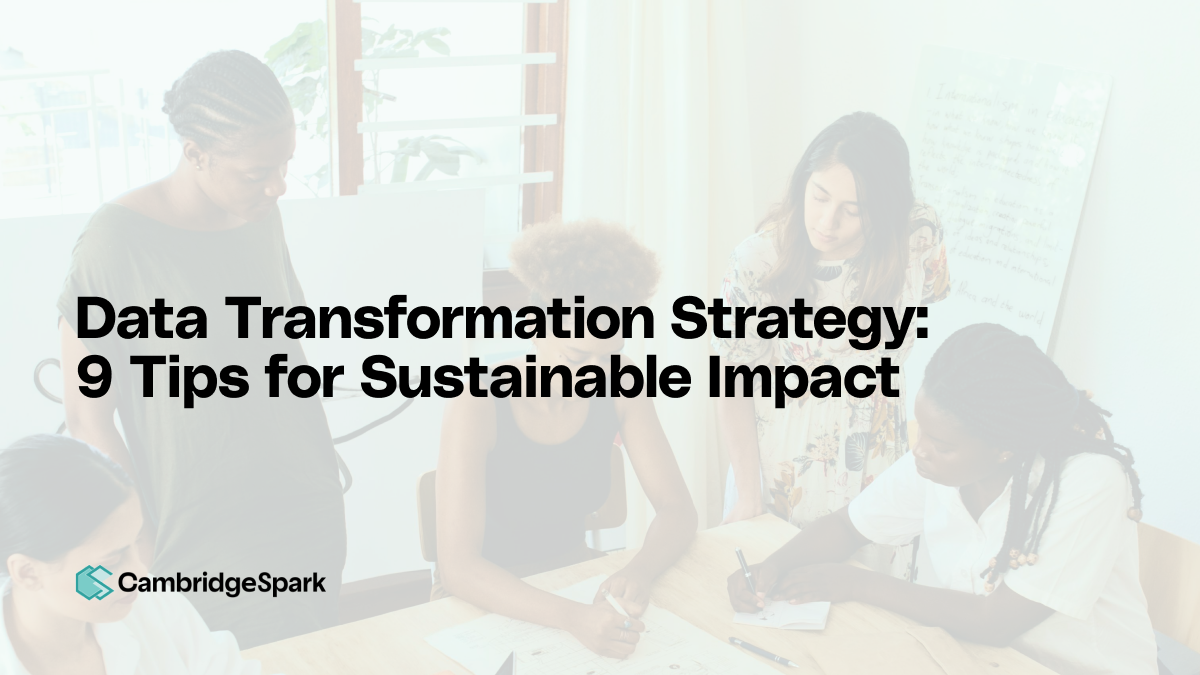
All businesses nowadays drown in data. Every second, your company generates massive volumes of information.
But here’s the real question: How much of this data is your business using effectively?
That's where most businesses struggle. Their real challenge lies in transforming that data into actionable insights and data-driven decisions.
To tackle this problem effectively, you need a robust data transformation strategy.
In this guide, we'll outline nine actionable tips that will empower your organisation to harness the full potential of data transformation.
By the end, you'll know how to define a clear data strategy, strengthen governance, and develop teams that thrive in the data-rich world.
1. Define a Clear Data Strategy
A clear data strategy is essential for successful digital transformation. It helps align your data work with your business goals, preventing chaos and ensuring that everyone is on the same page.
Think of it as a map of your current data landscape, covering datasets, technology, and team skills. This map lets you identify gaps and opportunities in how you use data.
Having a dedicated leader, like a Chief Data Officer, can guide this strategy. This person is responsible for overseeing data projects and making sure the entire organisation is working towards the same goal.
A strong data strategy also helps improve the way your business operates. It should include governance models, principles for data management, and a common vocabulary that everyone understands.
To create an effective data transformation strategy, focus on these key components:
1. Align with business goals
2. Evaluate your current data setup
3. Appoint dedicated data leadership
4. Integrate new initiatives with existing operations
5. Establish clear governance frameworks
This kind of strategy not only provides direction but also empowers your team. It creates an environment where using data leads to informed decisions, giving your business a lasting competitive advantage.
2. Foster a Data-Driven Culture
For any data transformation to succeed, you need your team to understand why data matters and how they can use it.
To develop a data-driven culture, everyone in the organisation should have access to the data relevant to them. When various team members can engage with data directly, decision-making becomes easier and more informed.
For example, your Sales team may use the data from your CRM to personalise their outreach. Your HR team, on the other hand, can address employee performance challenges.
This leads to actionable insights that help meet business goals and maximise the benefits of technology investments through enhanced analytics.
To execute a successful transformation, involve everyone in the process. When all employees understand why data matters and how to use it, it creates a strong push toward adopting data-driven practices.
3. Upskill Your Team
Investing in upskilling activities, such as apprenticeships or skill bootcamps, can significantly enhance your team's ability to utilise data effectively.
Providing your employees with the training they need to interpret and analyse data equips them with the tools to make informed decisions and derive valuable insights.
Consider implementing structured training programs that cover essential data analysis skills, data visualisation tools, and statistical methods.
“I had researched other providers of apprenticeships. But the thing that stood out for me with Cambridge Spark was the focus on business. You can apply data science in many different areas. But with Cambridge Spark, they will help you directly apply your learning to solve real problems in your business.”
Workshops led by experts in data science can further foster an understanding of advanced concepts and technologies, such as machine learning and artificial intelligence.
By upgrading the skills of your team members, you not only empower them to leverage data but also demonstrate the organisation’s commitment to employee growth.
This investment can result in higher job satisfaction and employee retention, as individuals feel more competent and valued in their roles.
4. Implement Strong Data Governance
Without a solid foundation for data governance, your organisation risks inaccuracies in decision-making or even violations of compliance regulations.
An effective data transformation strategy should be flexible and regularly updated. It needs to adapt to changing market trends and business needs.
Here are the critical components of a robust data governance strategy:
Data Stewardship
Data stewardship involves assigning dedicated personnel to oversee data assets and ensure compliance with governance policies.
These stewards are responsible for maintaining data quality standards, identifying data anomalies, and advocating for best practices across the organisation.
Data Quality Management
Establish robust metrics to assess data quality across various dimensions, such as completeness, consistency, and timeliness.
Regular audits, along with automated cleansing processes, can help identify and rectify data issues, ensuring that your transformation initiatives rely on high-quality information.
Data Accessibility and Security
While data should be easily accessible to authorised users, you should also adequately protect it against unauthorised access and breaches.
Establish a tiered permission structure that balances accessibility with security, allowing relevant stakeholders to access the data they need while safeguarding sensitive information.
Implement security protocols such as encryption and multi-factor authentication to enhance your organisation's overall data protection efforts.
Policy Development
Develop comprehensive data governance policies that outline procedures around data usage, sharing, storage, and disposal.
These policies should address compliance with legal requirements, including privacy regulations and industry standards.
Engage stakeholders from various departments to ensure that the policies reflect the diverse needs and realities of your organisation and remain flexible enough to accommodate future changes in technology and data practices.
Data Governance Essentials
- Data Governance Framework: Avoids Wheel of Chaos
- Technology Investments: Purposeful with clear business outcomes
- Strategy Evolution: Periodic revisits aligned with trends and priorities
- Operational Model: Clear checkpoints, IT and business collaboration
- Architecture Review Board: Monitors standards, guides changes
- Communication: Clear, consistent, vital for informed decisions
5. Utilise Agile Methodologies
Using Agile methods in your data strategy is vital for allowing your project to respond to changes in the business environment quickly.
Agile methods help organisations gain value through regular, small successes. These quick wins build skills and establish new practices throughout the transformation.
Agile encourages input from people at all levels of your organisation. This creates a data strategy that incorporates a variety of ideas and perspectives.
This flexibility enables organisations to achieve their data goals more effectively, making technology investments more worthwhile and providing practical insights that align with business objectives.
For Agile to be effective, it’s essential to have strong support from leadership and clear guidelines.
These elements ensure that everyone stays focused on the overall goals and follows Agile’s core principles, fostering a culture of continuous improvement and data-driven decision-making.
6. Break Down Silos and Go Beyond Traditional Data Teams
Integration across departments guarantees a robust transformation process that is more than the sum of its parts.
This unity forms a digital transformation project that is resilient, resourceful, and rigged for success.
When different departments—such as marketing, finance, operations, and customer service—collaborate, they offer valuable perspectives that improve how your business uses data. This cooperation leads to better insights from technology investments, aligns with overall business goals, and enhances customer experiences.
Key Benefits of Inclusive Data Transformation
- Better Understanding of Business Needs: Involving multiple departments helps everyone grasp what the business really needs.
- Smarter Decisions: Insights from different areas ensure that decisions are based on a comprehensive view of the company's data.
- Seamless Integration: Participation from all departments allows for easier data integration, making internal processes more efficient.
- Stronger Competitive Position: A united approach to data initiatives helps the company use advanced analytics and insights to outperform competitors.
7. Recognising Key Data Transformation Methods
A successful data transformation strategy relies on the effective application of various techniques that enhance data quality and usability. Three key techniques that play a pivotal role in the transformation process are:
Data Cleaning
Data cleaning involves identifying and rectifying inaccuracies or inconsistencies in the dataset to ensure that the information is reliable and relevant.
This technique addresses issues such as:
- Missing values
- Duplicate records
- Incorrect formats
All of which can significantly skew analytics and decision-making processes.
Robust data cleaning practices help you enhance the accuracy of their data, yielding higher quality insights that drive informed decisions.
Data Normalisation
Data normalisation means adjusting values within the dataset to a standard scale without distorting differences in the ranges of values.
For instance, normalising sales data from various regions can help organisations assess performance on an equal basis, enabling more accurate comparisons and trend analysis.
Normalisation not only enhances data integrity but also improves the performance of machine learning models by ensuring they are not biased towards certain data distributions.
Feature Engineering
Feature engineering is the process of using domain knowledge to select and transform variables into features that are more suitable for modelling.
This technique enhances the predictive power of machine learning algorithms by creating meaningful input variables from raw data.
By combining, decomposing, or recreating features, organisations can derive richer insights, which lead to more accurate predictions.
Effective feature engineering can facilitate improved model performance, enabling businesses to anticipate trends and make proactive decisions.
8. Ensuring Scalability and Performance Optimization
An effective data transformation strategy must also focus on scalability and performance optimisation.
As organisations grow and their data needs evolve, it's crucial to ensure that the chosen methods and technologies can handle increasing complexity and data volumes.
Here are three important considerations:
Infrastructure Readiness
Before implementing any data transformation strategies, organisations should evaluate their existing infrastructure.
This includes assessing:
- Hardware capabilities
- Cloud resources
- Data storage systems
Scalable cloud solutions can provide a flexible and cost-effective way to accommodate fluctuating data loads.
Continuous Monitoring and Adjustment
Data transformation is not a one-time process. It requires ongoing monitoring and adjustments to accommodate new data sources, changing business needs, and emerging technologies.
Establishing key performance indicators (KPIs) allows organisations to evaluate the effectiveness of their transformation strategies continuously.
This iterative approach enables teams to identify areas for improvement, pivot when necessary, and ensure that the data remains accurate, relevant, and usable.
Automation and Tool Integration
Leveraging automation tools can significantly enhance the efficiency of data transformation strategies. By automating repetitive tasks such as data cleaning and normalisation, organisations can devote more time and resources to higher-level analysis and strategic decision-making.
Automation not only speeds up the transformation process but also reduces the likelihood of human error, resulting in more reliable data outputs.
Here are a few ways to integrate automation into your data transformation strategy:
1. Data Integration Platforms
Utilising data integration platforms like Apache NiFi or Talend allows organisations to streamline the flow of data from various sources into a centralised system.
These platforms often provide visual interfaces for mapping data transformations, making it easier to set up and manage complex workflows without extensive coding.
2. ETL (Extract, Transform, Load) Processes
Implementing ETL tools such as Informatica, Microsoft SQL Server Integration Services (SSIS), or AWS Glue can automate the extraction of data from different sources, apply transformation rules, and load the processed data into data warehouses or analytical databases.
This automation not only saves time but also ensures that data is consistently prepared for analysis.
3. Machine Learning for Predictive Transformations
Leveraging machine learning algorithms to automate feature selection and transformation can provide insights into the most impactful variables for your specific analyses.
By using automated models, organisations can adjust transformations based on patterns and trends in the data without manual intervention.
9. Measuring Success with Key Performance Metrics
According to McKinsey's report, successful transformations realise only 67% of their financial potential, with significant value lost during target-setting and implementation.
This is why it's crucial to establish relevant KPIs that align with your organisation's goals.
Tracking these metrics will allow you to evaluate the success of your transformations and make data-driven decisions. Here are several KPIs to consider:
1. Data Quality Metrics
Assessing the quality of your data is fundamental. Metrics such as:
- Data Accuracy
- Completeness
- Consistency
- Timeliness
It will provide insights into the effectiveness of the transformation process.
A higher quality score indicates that the transformed data is reliable and can be used confidently in analytics and reporting.
2. Transformation Time
Monitoring the time it takes to complete data transformations is critical for evaluating the efficiency of your workflow.
By analysing transformation time, organisations can identify bottlenecks in the process and streamline operations to improve speed without sacrificing quality.
3. User Engagement and Adoption Rates
Understanding how frequently your team utilises the transformed data can offer insights into its relevance and usability.
High engagement rates suggest that the data successfully meets the needs of its users.
Low adoption might indicate that additional training or modifications are required to enhance usability.
4. Cost Savings and ROI
Analysing the cost savings resulting from your data transformation efforts will help you understand the actual value of your investment.
By measuring the return on investment (ROI) of your data transformation initiatives, organisations can ascertain whether the financial and operational resources allocated to these projects yield sufficient benefits.
Begin by identifying specific areas where data transformation has led to cost reductions.
For example, if automated reporting has replaced manual processes, quantify the reduction in labour costs and time savings.
This can be achieved through comprehensive analysis and comparison of pre- and post-transformation expenses.
If you need a more specific example, read how Visa's Imran Ayad leveraged data transformation to save 25-40 hours per client meeting through automated reporting.
Increased Revenue Opportunities
Additionally, data transformation can lead to enhanced business intelligence, which provides insights into new revenue opportunities. Track metrics related to:
- Sales growth
- Customer acquisition costs
- Market penetration rates
Before and after implementing transformations to assess the overall financial impact.
Comprehensive ROI Calculation
To calculate ROI, use the following formula:
Where net profit accounts for any increase in revenue minus ongoing operational costs related to the data transformation. A positive ROI indicates that your transformation strategy is contributing positively to the business's bottom line.
However, remember that ROI is not static. You should continually assess it over time. As your organisation evolves and external market conditions fluctuate, you may need adjustments to reflect the effectiveness of your transformation efforts accurately.
Qualitative Benefits
While quantitative measurements of ROI provide a clear financial picture, don’t overlook the qualitative benefits that data transformation can bring.
Improvements in decision-making processes, enhanced customer satisfaction, and better collaboration across departments can lead to long-term gains that are sometimes difficult to measure in numerical terms.
Conduct surveys and gather feedback from stakeholders to gauge the impact on organisational culture and innovation as a result of data transformation.
What is a data transformation strategy?
As organisations embark on their data transformation journeys, it’s essential to articulate what a data transformation strategy entails.
At its core, a data transformation strategy is a comprehensive approach that identifies how an organisation collects, processes, and utilises data to achieve its business objectives.
It encompasses various components, including technology, processes, and people, and integrates them into a cohesive framework that is agile and scalable across different organisational layers.
Components of a Data Transformation Strategy
- Data Governance: Establishing clear policies and standards for data management ensures consistency, accuracy, and security. Effective governance outlines responsibilities for data ownership, quality control measures, and compliance with regulations, which is integral for maintaining trust in the data being utilised.
- Technology Infrastructure: Modern data transformation requires a robust technology stack. From cloud storage solutions for scalability to advanced analytics tools for real-time insights, organisations must invest in technologies that facilitate data processing and visualisation. Consider adopting tools that support automation, machine learning, and artificial intelligence to enhance data capabilities.
- Change Management: Driving a successful data transformation requires more than just technology; it necessitates cultural change within the organisation. Engage employees by providing training and resources that empower them to use data effectively. Transparent communication about the transformation is crucial for fostering a culture that embraces change and innovation. Employees should be encouraged to embrace new technologies and methodologies, thus creating a more data-driven environment.
- Data Quality Management: High-quality data is the bedrock of any successful transformation effort. Implementing stringent data quality management practices ensures that the data is accurate, complete, and relevant for decision-making. Regular data cleansing, validation, and enrichment processes help maintain the integrity of data while also preventing erroneous insights that could adversely impact business decisions.
- Analytics and Reporting: A data transformation strategy must include a clear roadmap for analytics and reporting. Organisations need to develop capabilities to not only analyse data but also visualise it in meaningful ways. Investing in data visualisation tools allows stakeholders to derive actionable insights quickly. Additionally, leveraging predictive analytics can empower organisations to anticipate trends, improve customer experiences, and refine operational efficiencies.
Why Is Data Transformation Important?
You might have heard the phrase "data is the new oil."
It perfectly describes the importance of using your business data effectively. Here's what empowering your team with the right ways to handle data gives you:
Unlocking Value from Data:
Many organisations generate vast amounts of data but lack the knowledge to derive meaningful insights from it.
Data transformation enables businesses to convert raw information into formats that are more usable and easily understandable.
According to research from the MIT Center for Digital Business, companies executing a digital transformation are 26% more profitable and enjoy a 12% higher market valuation than their industry peers.
This conversion process is crucial for uncovering trends, patterns, and actionable insights that can drive informed decision-making.
Enhanced Decision-Making:
A well-defined data transformation strategy allows organisations to move beyond intuition-based decisions and towards data-driven decision-making that can lead to increased efficiency and effectiveness. By having a clear picture of metrics and KPIs, businesses can respond proactively to changes in the market or internal operations.
Improved Data Quality:
Transforming data not only involves converting formats but also entails cleaning and validating the data. Ensuring high-quality data is fundamental for reliable analytics, as poor-quality data can lead to skewed results and flawed business strategies.
Agility and Responsiveness:
A robust data transformation strategy allows organisations to stay ahead of the competition by enabling quick adaptations to market changes.
With the right processes in place, businesses can rapidly analyse data to identify emerging trends, shifting customer preferences, or operational bottlenecks.
This agility is crucial not only for survival but also for taking advantage of new opportunities as they arise.
Conclusion
Data overflows the business world. Having a solid data transformation strategy is crucial for any organisation looking to make a real impact. By tapping into the value of their data, businesses can make better decisions, improve data quality, and react swiftly to changes in the market.
The nine tips in this article will help teams view data as a resource that drives innovation and growth rather than just a byproduct of daily activities. Companies that focus on effective data transformation will stay ahead of the competition and succeed in a data-driven environment.
The journey to success is all about turning insights into actions—make sure your data transformation efforts lead to real results.
If you're interested in taking your team's data and AI skills further, check out Cambridge Spark's Apprenticeship Offer.
-1.png)
Enquire now
Fill out the following form and we’ll contact you within one business day to discuss and answer any questions you have about the programme. We look forward to speaking with you.